Quantum computing for
Earth Observation
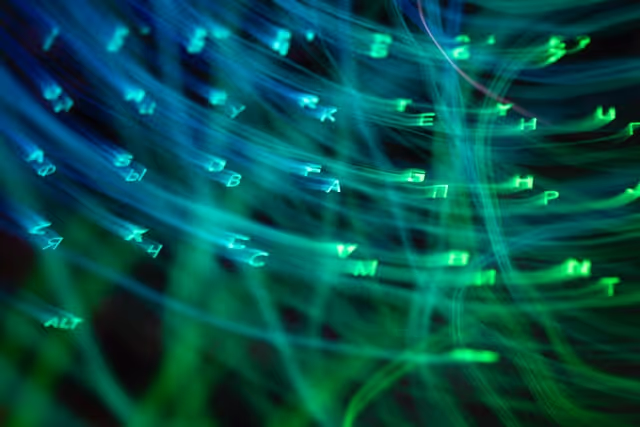
Quantum Computing in Remote Sensing and Earth Observation
The lecture series aims to provide a foundational introduction to quantum computing, tailored for non-specialists. It begins with a presentation of the basics of finite-dimensional quantum mechanics and introduces fundamental concepts such as Hilbert space, operators, quantum states, and measurement. Building upon this essential groundwork, the series delves into the core principles of quantum computation. Topics covered include an overview of existing models of quantum computation, architectures of quantum devices, and the fundamental components of universal quantum computation models, including gates and circuits. Additionally, the lectures offer a concise review of renowned quantum computing algorithms known for their proven efficiency compared to classical counterparts, and an overview of the current quantum computing landscape and a discussion on future prospects in the field. Transitioning to practical applications, the series introduces quantum machine learning, focusing on quantum kernels and quantum neural networks. Through hands-on sessions, co-organized with IBM, students will have the opportunity to experiment with basic concepts in quantum computation, design and implement quantum machine learning models with Qiskit.
Learning Outcomes
- Grasp the basic principles underlying quantum mechanics and quantum computing
- Understand the advantages and limitations of quantum computation, enabling them to navigate through various terms and concepts in the field
- Comprehend the design and real-life applications of quantum kernels and quantum neural networks
Agenda
Introduction to quantum computation
- Basic notions and postulates of quantum mechanics
- Measurement
- Introduction of quantum gates and circuits
- Computational paradigms and quantum computing hardware technologies
Quantum algorithms
- Logic behind quantum algorithms
- Advanced circuits
- Review of main algorithms
Hands-on session 1: Qiskit
- Introduction to Qiskit
- Basic gates implementation, state manipulation
- Experiments with quantum circuits
- Noise and overview of the available simulators
- Introduction to IBM Quantum Learning
Quantum machine learning
- Introduction to quantum machine learning theory
- Quantum kernels
- Quantum neural networks
Hands-on session 2: quantum machine learning for satellite data analysis with Qiskit
- Implementation of quantum machine learning models with Qiskit
- Application to Earth observation use cases
Instructors

Artur Miroszewski
Biography:
Artur Miroszewski is a postdoctoral researcher at Jagiellonian University. He obtained his Ph.D. in 2021 from the National Centre for Nuclear Research, Warsaw, Poland, in the field of theoretical physics. His doctoral thesis investigated the potential existence of quantum gravitational effects in the early universe, proposing a primordial singularity avoidance scenario known as the Big Bounce. His research also explored possible observational signatures of this scenario within the gravitational waves spectrum. Currently, Artur is actively involved in a European Space Agency project focusing on the exploration of quantum machine learning applications for satellite data analysis. His primary focus revolves around the utilization of quantum kernel methods for classification tasks.

Francesco Mauro
Biography:
Francesco Mauro received the Master's degree (with honors and career mention) in Electronic Engineering for Automation and Telecommunications in 2022 from the University of Sannio, Benevento, Italy, where he is currently pursuing a Ph.D. degree. He is also a Visiting Researcher at the Φ-lab, European Space Agency, Frascati, Italy, where he collaborates on research related to Quantum Machine Learning applied to Earth Observation. He has co-authored several papers in the field of remote sensing and has presented his work at reputed international conferences. He received the Best Poster Award at the IEEE GRSS IADF School 2024. His research interests include remote sensing and satellite data analysis, as well as Artificial Intelligence and Quantum Machine Learning techniques for Earth Observation. Since 2024, he has been serving as the IEEE GRSS Chapter Coordinator for Region 8.
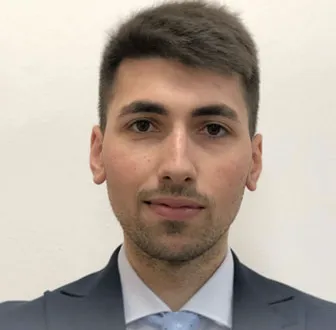
Alessandro Sebastianelli
Biography:
Alessandro Sebastianelli received the degree (cum laude) in electronic engineering for automation and telecommunications from the University of Sannio, Benevento, Italy, in 2019, where he also pursued the Ph.D. degree in Information Technologies for Engineering.
His research topics mainly focus on remote sensing and satellite data analysis, artificial intelligence (AI) techniques for Earth observation, data fusion and quantum machine learning. He has co authored several articles to reputed journals and conferences for the sector of remote sensing. Ha has been a Visiting Researcher with Φ-lab, European Space Agency ESA/European Space Research Institute ESRIN. He has won an ESA OSIP proposal in August 2020. He received an IEEE award for one the best the thesis in geoscience and remote sensing.
Currently he works as Research Fellow in Quantum Computing for Earth Observation at the ESA Φ-lab.
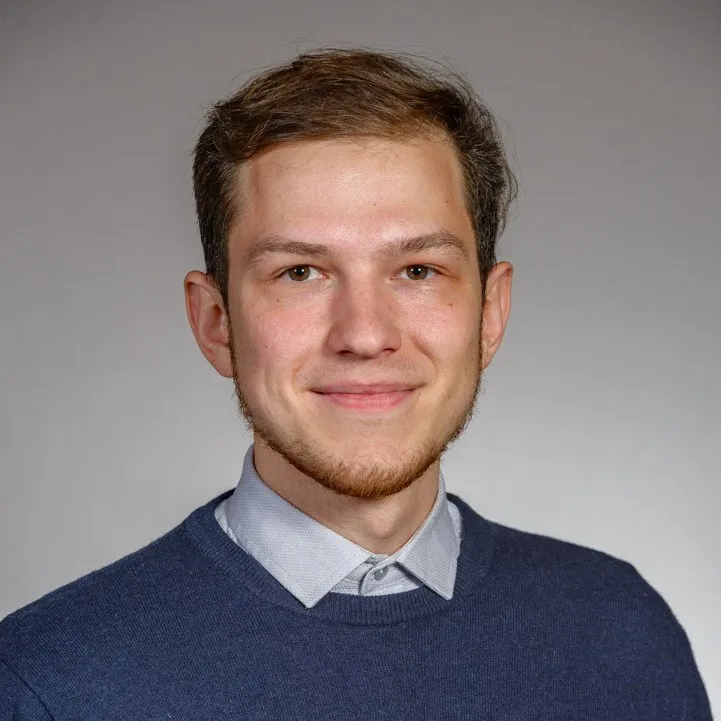
Amer Delilbasic
Biography:
Amer Delilbasic received his Bachelor and Master degree (cum laude) in Information and Communication Engineering from the University of Trento, Italy, in 2019 and 2021 respectively. He is currently pursuing his PhD in Computational Engineering at the Jülich Supercomputing Centre, Germany, and the University of Iceland, Iceland.
His research is mainly focused on machine learning and optimization based on quantum computing and high-performance computing for Earth observation. He has co-authored several articles to reputed journals and conferences for the sector of remote sensing. He has been a Visiting Researcher with Φ-lab, European Space Agency ESA/European Space Research Institute ESRIN. He has won an ESA OSIP proposal in 2021.

Silvia Liberata Ullo
Biography:
Silvia Liberata Ullo, IEEE Senior Member, President of IEEE AES Italy Chapter, Europe Liaison for IEEE GRSS, Industry Liaison for IEEE Joint ComSoc/VTS Italy Chapter, National Referent for FIDAPA BPW Italy Science and Technology Task Force (2019-2021). Member of the Image Analysis and Data Fusion Technical Committee (IADF TC) of the IEEE Geoscience and Remote Sensing Society (GRSS). Visiting Professor at ESA Phi-Lab. Editor in Chief for IET Image Processing. Graduated with laude in 1989 in Electronic Engineering at the University of Naples (Italy), pursued the M.Sc. in Management at MIT (Massachusetts Institute of Technology), USA, in 1992. Researcher since 2004 at the University of Sannio, Benevento (Italy). Member of Academic Senate and PhD Professors’ Board. Courses: Signal theory and elaboration, probability and Signals, Telecommunication networks (Bachelor program); Earth monitoring and mission analysis Lab (Master program), Optical and radar remote sensing (Ph.D. program). Authored 90+ research papers, co-authored many book chapters and served as editor of two books. Associate Editor of relevant journals (IEEE TGRS, JSTARS, GRSL and others). Guest Editor of many special issues. Research interests: signal processing, radar systems, sensor networks, smart grids, remote sensing, satellite data analysis, machine learning and quantum ML.